Next-gen infrastructure asset management technologies are critical to improving the margins of any operations. When it comes to industries that rely on expensive assets and infrastructures to enable operations, keeping assets functioning in top performance is critical to the bottom-line.
Planned Maintenance Is ‘Old School’
Planned-maintenance activities have been the core of the infrastructure asset management regime for as long as anyone can remember. Typically recommended by the manufacturers of equipment, planned maintenance activities provide prescribed usage parameters measured in hours in service, cycle count, or the number of miles/kilometers to determine when an asset should be maintained.
While planned maintenance activities are an integral part of an asset management routine, it is not considered state-of-the-art. Planning maintenance lacks context and time-sensitive health data derived from the specific assets on location.
Condition-Based Maintenance Lacks Predictive Capabilities
Condition-based maintenance at its most basic level uses a pre-determined threshold for a given asset or asset class and, based on some simple logic, initiates an action(s). Actions may include automatically opening a maintenance work-order to repair or replace an identified asset.
This approach is considered relatively basic-if threshold triggered, then do something. Of course, this logic can be expanded and become more complex. Still, the essence of this type of condition-based maintenance approach is reactionary in nature and cannot predict failures.
So, what is more stat-of-the-art in infrastructure asset management? It starts with understanding the digital replica concept, or as some like to refer to it, the ‘digital twin’ of the assets.
The Infrastructure Digital Replica / Twin
Every modern product manager knows the value of collecting health data of products in the ‘wild.’ The feedback loop for product performance in use in the field is essential to understanding if the design is performing as intended.
The increased proliferation of low-cost sensors coupled with wireless technology and cheap data storage has enabled an entirely new reality concerning asset management. This new reality means that many of the field assets are ‘digitized’ because they can regularly report on how they are performing, and by regularly, I mean in near-to-realtime.
By adding precise design requirements to the assets’ procurement process, asset owners can place the necessary building blocks for the digitalization of assets and introduce a digital replica that can then be harvested for additional value.
The Digital Replica Of Assets Holds Value
As digitization continues to blur the lines between physical maintenance and the maintenance of the physical asset’s digital replica, the amount of data gathered about the asset has increased exponentially. For example, performance data in context with other related factors such as the surrounding environmental conditions are now being collected by asset manufacturers to feedback into product development.
However, product development should not hold sole exclusivity to this data, as Operations and Maintenance (O&M) personnel are beginning to recognize a new gold mine of opportunity which, if managed intelligently, can add gains of 10 to 20% asset utilization improvements. These are big numbers that cannot be ignored, as it represents direct competitive advantages and improvements to the bottom-line.

There are powerful economic incentives to leverage real-time data and predictive maintenance. Companies can benefit from reduced costs, open new revenue streams, extend equipment life and increase production capacity.
-Martin Provencher, OSIsoft | April 16, 2020
Examples in Industry
A recent article was written over at Mining.Com, A guide to predictive maintenance for the smart mine, highlights some key elements required to move beyond historical maintenance approaches of reactive, planned/preventative, and condition-based maintenance regimes, and includes:
- Establish an operational data infrastructure
- Enhance and contextualize data
- Implement condition-based maintenance
- Implement Predictive Maintenance 4.0
AI helps unlock value from Digitalization 4+
New Artificial Intelligence (AI) applications, and more specifically, adding deep learning into the data-to-analysis workflow pipeline, will continue to augment the human experience. These AI-enabled tools are already helping to identify anomalies and patterns in the asset data hidden to the human eye but hidden in plain sight of the algorithms.
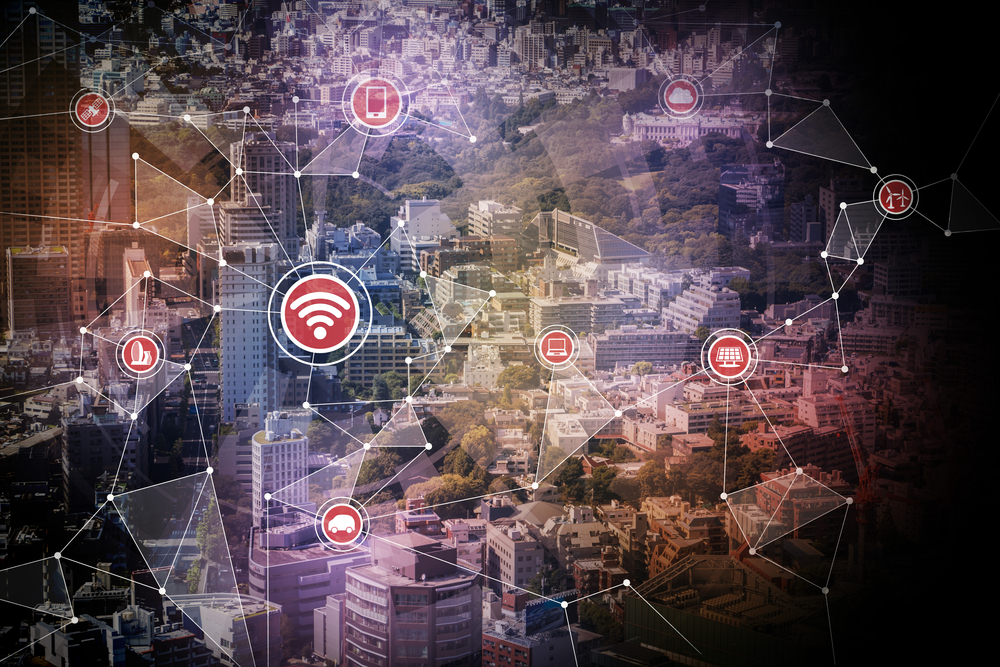
We will begin to explore more on the topic of Applied AI in the field of transportation systems in future articles and look forward to updating our readers as the field of examples continues to expand.
What is the role of asset management?
To optimize the Return on Investment (ROI).
What are The types of Transit asset management?
Transit Asset Management is primarily associated with the governance, optimization and maintenance of the transit assets and associated infrastructures. Types of maintenance regimes include: Planned Maintenance, Conditions-Based Maintenance, Predictive-Maintenance, and Prognostic Maintenance.
What Does an Asset Manager Do?
Ensure that there is a strategy and governance in place to continually optimize an asset’s probability to achieve maximum return on investment.